The Difference Between Decision Making and Making Predictions
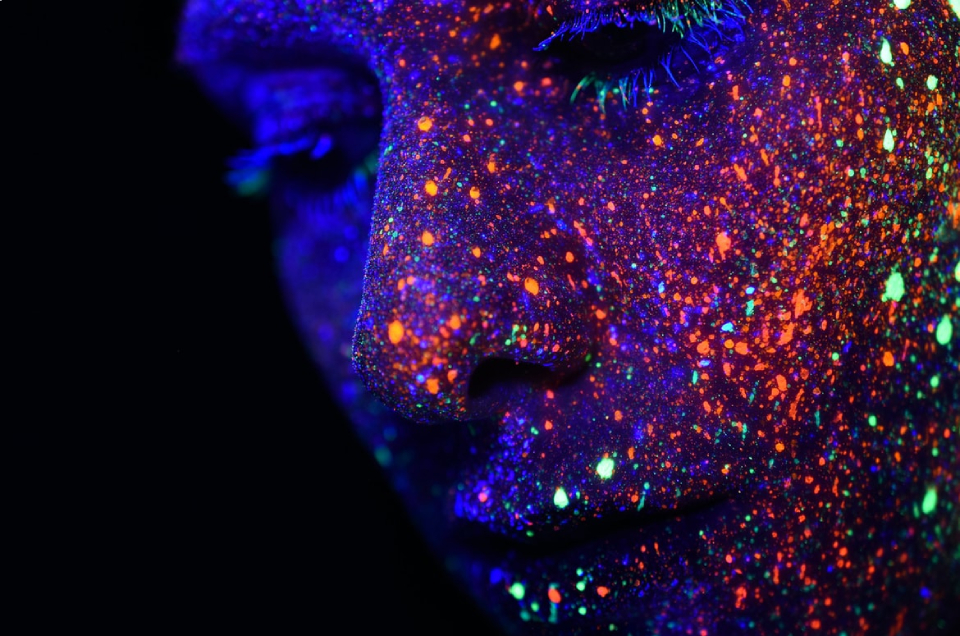
Prescriptive analytics is a popular term that is widely mentioned across various disciplines and businesses and often discussed on blog posts. In this article, our goal is to allow the reader to walk away with a clear distinction between prescriptive and predictive analytics.In general, the goal of prescriptive analytics is to sequentially provide the user, such as a CEO, CFO or COO, with a plan of action that is designed to maximize or minimize some specified target metric. A common example of this would be profit maximization for a given business. As you can imagine, there are many drivers that affect the profitability of an organization. A company is able to control some of these drivers (i.e., we can call these control factors) but other elements are completely outside control. Therefore, prescriptive analytics aims to provide a set of instructions to decision-makers such that for each decision made with respect to any control factors, the higher the probability that profit is maximized.
In contrast, consider the situation where a user would like to forecast a specified target over a period of time. This task is often referred to as predictive analytics. Modern day predictive analytics is often used in fields such as engineering design, drug discovery, weather forecasting, stock price prediction, and even cancer detection. The goal here is to provide the user with a crystal ball that opens a window into the unexplored. One of the most important factors when creating a predictive model is the quality of the dataset it is fed. The more reliable the data, the more accurate the predictions will be. As a result, one of the most valuable assets that a business has is its ability to store their data. In some cases this may seem like a headache, but it is worthwhile as this collection of data will allow a business to construct the most accurate model possible.
Now let’s distinguish between prescriptive and predictive analytics. Keep in mind that comparing the two is akin to comparing apples and oranges. Recall, prescriptive analytics is designed to generate a set of decisions that can be presented to the user. In order to do this, we actually utilize predictive models to drive this search since we do not have an exact model that describes reality. Thus predictive models are one of the main components used in the prescriptive analytics methodology. In short, the steps involved in prescriptive analytics include:
- Obtain a dataset of interest and specify a target variable.
- Train a predictive model that can describe the response of the specified target.
- Locate a point of interest (i.e., generate a decision) by optimizing the predictive model.
- Carry out this decision and obtain a new observation for the target.
- Augment your dataset with this new information.
- Go back to step 1.
Since we are optimizing the predictive model in step 3., over time the decisions that the prescriptive system generates will begin to maximize the target of interest (which is often profit or revenue). As we keep repeating these steps over a number of months or years, you will expect to observe a realized improvement in your target.
To wrap up, here’s an illustrative example that compares the two strategies in a practical setting. Consider the controversial topic of climate change. In this setting we have variables that we can control (e.g., CO2 emissions, pollution, etc) and variables that are outside our control (such as weather patterns, solar intensity, etc). Suppose our goal is to reduce the increase in the average global temperature. If we were to take the predictive analytics approach, we would be able to predict what the average global temperature will be in a given year based on historical data. However, this does not help us with our goal. If we were to take a prescriptive analytics approach, we would be able to leverage predictive machine learning models to generate decisions that help us to achieve our goals. Over time, we would begin to record the response to our actions, update our datasets and continue to make decisions that lead us closer to our goal along each step of the way.
In the context of businesses that want to use prescriptive analytics to increase their profitability, prescriptive analytics can be used as a guide to help drive the decisions that bring you closer to your goals. Predictive analytics is one of the powerful tools that you will have at your disposal, but this tool alone will not be able to provide you with the insight you need to optimize your targets.